Radiomics Shows Promise for Assessing the Efficacy of Cancer Treatments
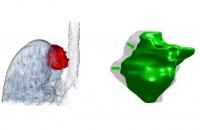
Left: In a 3D rendering of a baseline CT-scan, the lung tumor is displayed in red. Right: The change in tumor volume and shape after systemic therapy. In this patient, radiomics quantified a decrease in tumor volume, a decrease in the invasion of tumor boundaries, and a decrease in tumor spatial heterogeneity which predicted a favorable outcome and response to systemic therapy.
New cancer therapies are providing oncologists with more treatment options—and as a result, more hope for cancer patients and their families. In addition to surgery and chemotherapy, a physician's toolbox now includes immunotherapy, biologics, and various targeted therapies.
This array of systemic therapies presents a new challenge: how can we assess which patient will respond to which therapy? For patients whose chosen treatments are not working, it is essential to answer this question as early in the treatment process as possible so that they can be switched to another therapy.
Scientists from the Department of Radiology are using artificial intelligence to extract a solution from standard-of-care CT scans. In research published in Clinical Cancer Research earlier this year, associate research scientist Laurent Dercle, MD, PhD, and colleagues from the Computational Image Analysis Laboratory—headed by Binsheng Zhao, DSc—studied clinical trials of three different cancer treatments in patients with non-small-cell lung cancer (NSCLC). They found that they could predict whether a therapy was working for individual patients after just two months of treatment.
“The purpose of this study was to train cutting-edge AI technologies to predict patients’ responses to treatment," Dercle explains, "allowing radiologists to deliver more accurate and reproducible predictions of treatment efficacy at an early stage of the disease."
The clinical trials Dercle and colleagues worked with involved experimental treatments for cancer. To measure the success of a treatment during the trial, radiologists measured CT scans taken at regular intervals. If a patient’s NSCLC tumors reduced in size and no new lesions appeared, the treatment was considered to be successful.
But the CT scans contain a wealth of visual information beyond tumor size and number of lesions, Dercle says, and his study focused on quantifying this additional information.
An expert radiologist can estimate whether a patient is responding to therapy by looking at factors like the shape, density, and texture. But because these judgements depend on clinical experience, they are subjective and may vary according to the individual looking at a scan. Even though waiting for tumor growth or new lesions can mean critical time is lost before the patient is switched to another treatment, clinical trials rely on these measurements because they are reproducible from one site to another.
The machine learning techniques used by Dercle and colleagues can objectively and reproducibly quantify aspects of visual information not previously taken into consideration, as well as patterns that are not discernable to the human eye. Dercle’s group focused on a few key features, including tumor volume, margins, shape, and heterogeneity, found in clinical CT scans of patients before and on first-treatment assessment with three different drugs: nivolumab (immunotherapy), docetaxel (chemotherapy), and gefitinib (targeted therapy). These images were made available for research by the clinical trial sponsors through a public-private partnership.
The Columbia researchers observed that an exponential increase in image features deciphering tumor volume, invasion of tumor boundaries, or tumor spatial heterogeneity were associated with treatment insensitivity and shorter overall survival. Using this set of features, known as a radiomic signature, they could predict individual outcomes to treatment earlier than traditional measurements of tumor size and lesion count.
"This form of personalization uses non-invasive technology and is far greater than anything we’ve had before in cancer treatment," says Lawrence Schwartz, MD, James Picker Professor of Radiology and chair of the Department of Radiology. "The aim is to personalize therapy for a patient using imaging biomarkers, to guide the oncologist to select the most appropriate therapy and modify it sooner if it is not working."
Their technique could be applied by clinicians to better guide the decision to continue or modify a patient’s therapy much earlier in the treatment process.
References
More information
The findings appear in “Identification of Non–Small Cell Lung Cancer Sensitive to Systemic Cancer Therapies Using Radiomics,” published March 20 in Clinical Cancer Research.
Additional authors: Matthew Fronheiser, Lin Lu, Shuyan Du, Wendy Hayes, David K. Leung, Amit Roy, Julia Wilkerson, Pingzhen Guo (Columbia University Irving Medical Center), Antonio T. Fojo (Columbia University Irving Medical Center), Lawrence H. Schwartz (Columbia University Irving Medical Center) and Binsheng Zhao (Columbia University Irving Medical Center).
What is Radiomics? Watch our short animated video.